Into the AI Trough of Disillusionment
TOM [00:00:01]: Hi, Nate.
NATE [00:00:03]: Hey, Tom.
TOM [00:00:05]: The two points of intersection of the moon's orbit and the ecliptic are called lunar nodes, or dragon points. End quote. The ascending node is the head of the dragon, the descending one its tail. Both points play an important part in the calculation of the calendar. They were used in classical astronomy, chiefly for the calculation of solar and lunar eclipses. It's a quote from a book called Alchemy of the Seasons, which I've been reading. For those watching this, there's an image of the dragon. Nice. And the dragon points. So I just want to add that when I used to play World of Warcraft, there was this thing called dragon kill points. And I got a lot of those, which sort of meant that I was supposed to get good gear when you're doing raids and whatnot. But apparently there's real dragon points, which happens when there's an eclipse. So if anybody got good raid gear on Monday during Totality, they should let us know.
NATE [00:01:16]: Yeah, we've got raid gear. We've got our Totality hats on. Welcome to the retort. I didn't laugh enough at these jokes, but we're going to talk about the many different hats that we wear. And mostly do this through talking about the apathy of open model releases. I no longer feel. Mistral released a new model last night, which is a large... Once they're in this 100 billion plus range, it really... What does that even mean? It's not easy for me to even fine tune these with the compute that I have access to. I think we figured out this Llama 70B thing, and we're well and truly in a mini rush before Llama 3 drops. I think in the same day yesterday. This is just coming to me, and it's filling Tom in, who's had busy other stuff recently. OpenAI released a new version of ChatGPT with almost no documentation. John Schulman did chime in to Twitter and be like, we're going to release a blog post with release notes soon. John is probably the most trustworthy OpenAI employee on Twitter. It's great to see him, and it's like set the record straight sometimes. Google made the Gemini 1 million context length model free to use and accessible to everyone at aisudio.google.com, where you can upload multiple gigabyte audio files and have it analyze the quote-unquote motion of the model. The information released a report saying that Llama 3 is coming quote next week or something. And then Google quote-unquote confirmed it. We're considering on YOLO dropping a new model at Allen AI to get out before Llama. It is well and truly hilarious chaos out here. And right before recording this, I was talking to the NTIA, who's trying to make sense of open source AI models so they can write a report to the president. And I don't think this is helping them figure this shit out. And I tried to explain to them that this is just how it's going to be. And some of the frameworks I have are thinking about OpenAI, like openness in AI as a word. But I think that's kind of it's like a reflection of what it means that a lot of the practitioners are kind of apathetic with the pace that's happening. It's like, I don't like I'll use these models when I can, but it's kind of baked into my assumptions now that things are going to keep getting better. And I'm just going to kind of ride it out for the long term. And that's where we're at. So it's still fun, but it's different.
TOM [00:03:49]: So yeah, I spent the last week getting ready for totality. I now live also in D.C. That's new for me. D.C. area. It's a little bit warmer here. Spring is nice. And my wife and I drove from D.C. to my cousin's wedding in northwest Ohio. Shout out to Spencerville, Ohio population. Something like 450 people. Or 20. Yeah.
NATE [00:04:21]: Why didn't Mistral release their new model during totality? That would have been great. Here's the Eclipse. Here's the special release. None of these things are serious to begin with.
TOM [00:04:33]: It does put things in perspective. It's interesting how, I mean, I was into, I care about this stuff just because I kind of dabble. I have a kind of hobbyist interest in astronomy and celestial stuff like that, you know, ever since I was a kid. On top of which, yeah, my cousin got married during it and right in the middle of the path of totality. So there was a double whammy there. And it was a very interesting experience because it was a contrast with this world that we talk about here just in the sense that there's this total loss of control that you just sort of feel when literally the celestial bodies align with each other. It has nothing to do with you. If there was no people on Earth, if there was no life on Earth, it would happen. I mean, some version of that eclipse is always happening somewhere because these planets always exist relative to the sun. And so it's really just a statement of our arbitrary relationship with the cosmos that we happen to have these experiences. And it just reminds you. I wouldn't describe it as like nihilistic. It's not like, oh, nothing matters and I don't matter. It's more like I'm really just and we're all just bearing witness to these literally cosmic forces that are going to play out no matter what we do. And our feelings about them are entirely. I mean, secondary is even still, I think, too much to describe what their significance is. They're just beside the point. And it's a really strong, for me, I guess, emotionally. It's a very interesting difference from AI, where I often feel like it's the opposite. And especially since CHAT2BT, it's been the opposite, where it's often our feelings, our expectations, our attention, our assignation of hype to the next model or the next. Because we do kind of treat these models, or we used to, kind of like eclipses. Like they just would break the internet or break Twitter, like whatever they dropped. And there was a kind of mini totality around their release. And I feel kind of liberated from that, having actually seen the physical forces aligned for the eight minutes of totality. I was on a farm, and you could see all the birds get confused. They were like buzzards flying around, being like, should I eat right now? Am I hungry? Because they thought it was dusk. And I was describing to Nate before we were recording. There's this interesting phenomenon where basically it's almost like a 360-degree sunset that happens. Because the sun is still technically in the sky, but light isn't hitting the earth anymore. And so the sunset effect is happening kind of all around you. So there's this kind of orange-ish burned effect on the horizon all around where I was standing. And also northwest Ohio is incredibly flat because of the ice age glaciers having just completely flattened it like a pancake. So you can see all around you against the horizon, this kind of sunset effect. And so it was cool. It was kind of mysterious, even though the physics of it are remarkably simple.
NATE [00:08:02]: Are there people that are not listening to retort the confused buzzards of the AI community?
TOM [00:08:10]: You know, it's funny. Once you understand the underlying mechanics of what's going on, what remains mysterious is not the thing itself because it's remarkably simple, right? The moon is just approximately the same size as the sun from our perspective. And so it's the only reason this whole event feels like anything to us. It's a complete happenstance, right? And I think there's something parallel where for me what was most interesting about the eclipse, I mean I was already indicating this, what was most stimulating for me was not the totality itself in the sky, but the effects that it was having on the terrestrial plane, right? So I described this sunset effect. It just got dark very quickly. It got colder very quickly. As I mentioned, the birds and the animals on this farm, the dogs started barking. It's confusing. It's mystifying. And it's mystifying not because the physics are complicated, but because our relationship with the cosmos is this, you know, we take it for granted. We rarely ever bring it to awareness. And suddenly God was basically poking a finger at us being like, now you're aware of these forces, these billiard balls that have been put around you.
NATE [00:09:38]: And you just have to revel in it. The three-body problem recently came out too, which was really timely on this. I started it.
TOM [00:09:48]: This is kind of a running joke for me, because ever since I joined the kind of AI world in, I guess, late 2016, I had like a running ticker of like every two months, some new person would be like, you have to read this book.
NATE [00:10:03]: I like it. I'm reading the second one.
TOM [00:10:05]: It's amazing. Oh, you're in the second one? I'm reading the second one.
NATE [00:10:08]: And I watched the Netflix show, which is opinionated, but I see why they did it. So most of the book happens in the internal dialogue of the main character. So in the show, they essentially split the main character into multiple people so they can talk. And then you have the dialogue. And I see why they did it. But they like Americanized it, because most Netflix viewers don't want to watch something that's all in Chinese. And it's like, okay, I understand. It's like a Netflix-sized TV. It's not an HBO show.
TOM [00:10:36]: I read that the show is, yeah, kind of a more globalized version of the story. Yeah. Yeah.
NATE [00:10:44]: I think it very fits into the mood of the whole Eclipse thing. It's a very out there story. I think thinking about like the Eclipse and stuff, it's like this is a hard transition, but it's like the Eclipse, the totality is really cool and everything. But it's like with these things that get so much hype in AI, it's really an open ecosystem, particularly. Like they're all going to under deliver. It's like they're going to get hyped up so much. Like Llama 3 at this point is totally set up to fully under deliver. There's no world where Llama 3 comes out and it over delivers on what people expect from it at this point, because it's just been so long put off. It's going to be a better model in some ways. And it's another step along this long journey of figuring out how far these kind of open models will go. And it just takes so long to figure out. Like this is what everyone said with the product overhang. We have to figure out how to use these models in the open. And it's almost like there's more work to be done there than there is that these open models getting that much better will do. But they will continue to keep unlocking things. It's like talking to one of my best friends who's doing a startup and he's like this new Cloud, Sonnet and Haiku models are really useful, but he doesn't have enough throughput. So essentially if it was an open model that was exactly the same, he could spin up the GPUs and it would be cheaper for him to do it himself. And he could actually do this in this product he's trying to deliver. So it's like this weird balance of the hype no longer delivering, but progress continuing. And these use cases are getting closer to actually working and things like this. So it's this kind of weird mixed bag of I'm apathetic to the new models. Like Grok came out from XAI, I didn't care. It's a good use case to test my frameworks for understanding openness. It's like they did no disclosure and it's not really accessible to anyone, but it is publicly available. And it's like, okay, that's a good data point, but it doesn't really matter. And this new Mistral model will matter to relatively few people because essentially this is going to come out of left field. But you need about 260 gigabytes of VRAM to run this in FP16, which is the most basic data format for storing the model in the memory of the GPU. A top end A100, H100 that you hear about has 80 gigabytes of RAM. So you need at least four GPUs to actually run this in a normal configuration of like a relatively well-known GPU that's just for inference. So like people are going to figure out how to run this locally, but it's just like kind of a pain. Like in order to train this, you're going to need multiple nodes of these really hard to get GPUs, which is just not that many people have access to it. It'll be a data point on some papers, but it's not like in the Overton window of what is like the scientific Overton window, which is what the vast majority of scientists who are actively understanding these things and driving the field forward can use. And if it's outside of that window, I have a hard time being as excited about this because my goal is to try to understand these things and to make the technology more transparent. But within the window, there's not as big of performance improvements by improving things within the window as well. So I see why it's like harder to create hype where you're like, we released another 7 billion or 30 billion parameter model that's just like slightly better. So it's kind of like mixed incentives, mixed storylines that just leave people sometimes confused, I would guess.
TOM [00:14:06]: I've tried to wait to bring this up as long as possible, but I feel like it's relevant now in this podcast, which is the, and I'm going to mess up the name, I have to look it up. I'm looking at the graph of technological hype or graph of inflated expectations.
NATE [00:14:21]: Oh, yes. The up and the down and the up again.
TOM [00:14:24]: Let me look it up just because I don't want to mislead exactly what the, because I think it's now an interesting, okay, sorry. There is a more formal name. Excuse me, I forgot this. I have the Wikipedia page in front of me right now.
NATE [00:14:40]: The Gartner Hype Cycle. Wow, this is great.
TOM [00:14:43]: Is that with a T? It is with a, it's Gartner. So yeah, G-A-R-T-N-E-R, which amusingly is not a man or a woman. It's just the name of an IT firm.
NATE [00:14:54]: Oh my gosh, this is amazing.
TOM [00:14:56]: It's fun. I didn't know that. That's amusing. I've seen this graph many times. So it's this hype cycle. So I'll just kind of qualitatively describe it. Briefly, you've got two axes, which are called time, which makes sense, then visibility, which is an amusing euphemism for hype, I guess. There's a rapid ascension along the visibility axis, which is called the peak of inflated expectations, which follows the initial technology trigger in our world. I guess that might be just something like, you know, technically, ChatGBT is an interface, but let's just say large language models or something like that. So it's a peak of inflated expectations. It's very rapid. Then there's an almost equally rapid fall into what's called the trough of disillusionment. It's kind of a pretentious name for it, basically.
NATE [00:15:46]: It's got to be our episode title.
TOM [00:15:48]: People just learn to, you know, not care so much. It's not just that, though. It's that you're repeatedly, I think it's this idea that you're kind of successively disappointed or frustrated.
NATE [00:16:01]: It's like, we're not there yet, but we might be in a few more months.
TOM [00:16:04]: It's important that it descends, but it doesn't go all the way back down to zero. The visibility is still there.
NATE [00:16:11]: I think it's going to get a long time before we hit this trough of disillusionment. Okay. I think we're just starting to come down into it.
TOM [00:16:21]: Yeah. That vines with what I was going to say.
NATE [00:16:24]: That's when the startups start dying. That's when the whole GPU bubble cracks a little bit. That's when all that happens, I think. The derivative is well shifted, I would guess.
TOM [00:16:40]: I've seen this graph discussed in the context of automated vehicles as well, which I think we're kind of farther. To the extent to which it's really technology specific, we're farther along, I think, on the automated vehicle front. The last two parts of this graph, which anybody can look at, after the trough of disillusionment, you have the slope of enlightenment is what it's called, which is the sort of somewhat more gradual increase in visibility over time. And then what's called the plateau of productivity.
NATE [00:17:11]: I think this will get rewritten from AI, just because there's so many AGI people that write the narratives of AI, they're going to be like, this plateau of productivity is not allowed in our news. Yeah.
TOM [00:17:23]: I think that's a good point. It's a fecund graph because it tells, what it's really doing is telling a story and it's telling a very different kind of story, certainly than the accelerationists who are just, I think, fundamentally allergic to the idea that there will be any kind of plateau or
NATE [00:17:43]: any kind of reduction. It's funny because the safety people also say this. It's not just the accelerationists. Some of the safety people do acknowledge the compute restrictions, where it's like, there's not going to be enough electricity going to data centers to allow for this exponential acceleration as things currently are. That's the hardest constraint, which I find honestly really wonderful that it's like, we just don't have enough power plants to make a singularity happen. I mean,
TOM [00:18:14]: right. I don't know if we've discussed this on the pod yet, but yes, there's been these statistics kind of bandied about that. It's something like to train the biggest models. Now you're already basically needing a grid, the size of like, or at least a grid whose ability to power a country is like we're on the level of like the Czech Republic or something like that in terms of like scale. And of course, you know, this is there's, there's a few different variables here that I'm collapsing for the sake of this conversation. But if you do believe that the size of these models is to put it mildly growing and to put it also mildly exponential, it's not really clear to what extent or how quickly that like slope of
NATE [00:18:59]: electricity generation contributes to disillusionment. I think if you tell people that our AI technology is limited by our power grid, they're just going to be like, that's a dumb, I want to joke about that. So I'll, I'll link to this in the show notes. It was an information piece that I was essentially like Microsoft and AI are plotting this like a hundred billion dollar supercomputer. And I think within it, they essentially say like the biggest problem is that you can't put this in one place. Cause if you were to put this in one place, it would take down any state's energy grid. So you have to figure out how to connect multiple data centers together because the power needs are like double digit percentages of any individual state's power usage, which is a huge, I'm given some crap to people that like all they do is complain about the climate impacts of AI. I think if you're working on the, like go work on that, if you're working on climate and AI, which is like how to make it so that our infrastructure is prepared for the gross capitalistic incentives coming to manipulate the power market just to train AI models. Like big, big tech has a lot of levers and I could see them doing some weird stuff. So I, I don't know how to access that, but maybe you'll be a journalist and poke around and like show up at the data center and be like, ha ha ha. I found you.
TOM [00:20:16]: A hundred percent. This is something that it it's, it's what I think it is a classic example of how regardless of like what you may think about the more far off thought experiments that have defined the safety discourse or parts of the acceleration is discourse. I genuinely believe this is one of the first that is already, we're already feeling the pressure of this. And it's, it's, it's not really surprising because it's so material, it's a materialist constraint on scale. And those have always been the most urgent, the most significant and the most transformative, regardless of what you may speculate about the technology in some abstract sense. It's always, even for you to test those speculations, the infrastructure capacity you would need to even get there is so demanding that it just contorts the existing politics, economics, resources, labor, whatever the resources that's at stake implicitly or explicitly becomes redefined.
NATE [00:21:24]: I think people are gaslighting most of the community when they talk about the like the AI hiring landscape. When in reality, most of it is like a resource procurement by big tech trying to get who like has access to the GPUs and can set up the GPUs in the right way is defining a lot of this in the background. Right. Like the talent obviously matters, but I think the talent is overblown because the people that matter are normally like a small subset, like some really small percentage are the people that drive a lot of this at the cutting edge. So I, it's like a lot of smart people in AI are saying this where it's like, it is a sort of resource problem. Like this era of AI is defined by how much compute people have and how much engineering capability they have to throw out a problem, but just, there's like, it's so different than the narrative that you're actually going to get.
TOM [00:22:11]: I mean, this is supposedly why Sam Altman is just kind of traveling the world, trying to get Saudi Arabia to build, you know, plants to make chips there or, you know, any number of other things. It's about global supply chains, really. When you can scale to infinity, that really just puts pressure on where the supply chains currently are. That doesn't mean we just automatically get to infinity. It means that the pressure points in the world economic system become, they start to buckle under the weight of those expectations.
NATE [00:22:43]: Has the infrastructure by your new home buckled under the weight of its own gravity with this large container ship?
TOM [00:22:51]: It's, oh yeah, the Baltimore thing. It's interesting. I haven't been directly impacted by it, although it's maybe tangentially because I did move, I guess a little like 10 days ago, I moved from the Upper West Side in Manhattan to, to the DC area. And so we did both my wife and I drove our car and also our movers took the same route that went presumably right by where that bridge was. That being said, pretty remarkable how there was some traffic, but it wasn't really, there was no like massive slowdown. This is probably long enough after the collapse that it didn't immediately impact me. But yeah, we're seeing that as well. I think, I think, yes, I think that's right. I mean, we also saw this in, how long was it since the, the ever given ship got stuck in the sewers?
NATE [00:23:47]: Evergreen?
TOM [00:23:48]: Evergreen, is that what it was?
NATE [00:23:50]: I think it was Evergreen. That was great memes.
TOM [00:23:52]: They got stuck in. Well, yeah, it's actually, I went down one of my weirder rabbit holes where I was like studying it over
NATE [00:24:00]: time. It was ever given.
TOM [00:24:02]: How it was.
NATE [00:24:03]: Yeah.
TOM [00:24:04]: It's a funny name.
NATE [00:24:05]: It is funny.
TOM [00:24:06]: Yes. There's a, there's a very interesting correlation over time of like the average size of these global shipping containers relative to the like size of the canals through which they have to move. And so we just take this for granted, but like the Panama canal is not a natural feature of Panama that had to be made. It was a huge, you guys know the palindrome, right? A man, a plan, a canal, Panama.
NATE [00:24:33]: Oh my God.
TOM [00:24:34]: Maybe that's just me. They said this about Teddy Roosevelt, I guess, because that's what sort of what one of his slogans was. And so I don't know why that was interesting for them to make a palindrome out of, but they decided to do that. And then of course that was, that was like America becoming an imperial power was like, look at what we can do. Look at how we can move earth and water. To open up the relationship between the Atlantic and the Pacific and the shipping routes. Of course, the British empire had Suez canal and that we're going to see this.
NATE [00:25:06]: AI is the thing we're going to see.
TOM [00:25:09]: I think we're already, we're already see it. Yeah.
NATE [00:25:12]: With all the safety institutes and the pressure to legislate open or not, it's like, it's really like if any countries step wrong and like shoot themselves in the face and then they're like now just out of the part, out of part of a discussion on open models. I mean, just recently I didn't read this, but there was this new bill proposed by, I think Senator Schumer's office that essentially would like restrict open anything because it was like, I think it was something along the lines of this isn't going to get passed, but along the lines of anyone training, a model needs to disclose all the copyrighted information and their training data like until the government. I was like, yeah, that's not going to happen. Like we're now watching this play out where the kind of guardrails for AI are set or not. I do think that it's kind of going to be that the European union tries to prop up Mistral. Mistral doesn't need propping up now, but in a few years they might like they're trying to playing. They're in a similar boat to cohere and it's impressive that they have kind of put themselves in the same conversation of cohere in such a short period of time. You get to give them credit for that and they're just kind of in the middle. Cohere is open sourcing some models now and getting good community recognition, but like what's the longterm plan is open AI just going to eat everything because they can mobilize more resources. They could set up more data centers and like that might just make them win. Like we were just talking about the resource allocation and yeah, like, like open AI and Google are winning at that race. Google is clearly winning ahead of open AI just because their background and building a large scale compute. Everyone in the middle, like anthropic Mistral cohere, we don't know what's going to happen with them. Inflection already kind of died. That's not even a real story, but like all these places eventually are going to run into barriers of being a real company and it's probably still going to be kind of this PowerPoint power problem. The government pipe problem up.
TOM [00:27:11]: It always turns into a power problem eventually. And it, I think it's just that there is a scale problem here. We don't know quite when,
NATE [00:27:20]: or this,
TOM [00:27:21]: I don't know quite when that's going to top out in terms of like, you know, how much compute can a woodchuck Chuck, if which could compute something, you know, of course people, there's been a lot of ink spilled and there will be a lot of ink spilled
NATE [00:27:35]: about that. I'm not,
TOM [00:27:37]: I'm not sure if anybody knows the answer to that. That's part of the mystery that we're, we're inhabiting. This might be a way for us to transition a little bit to something else that we wanted to touch on, which is how we're in the middle of this could be connected to that graph point. Actually, that one reason we've got these expectations, maybe deflating slightly or people are, are shifting their emotional energy around what the latest model does or doesn't mean is that it seems like risk profiles are now becoming increasingly understood as not just referring to models in some abstract sense, but to the use cases for these models. And there, I think are some dimensions along which that happens that people really haven't thought much about yet or haven't written much about yet, or we're just still analytically a little bit mixed up about that. Cause to some degree that might be model specific or here ability specific, but it's really more just about who's using us to do what and why and what constraints are being put on that, either in terms of like literal algorithmic constraints or more in terms of like API, like level stuff or any number of other,
NATE [00:28:47]: you know,
TOM [00:28:48]: layers to matter.
NATE [00:28:51]: Yeah.
TOM [00:28:52]: So I think that this,
NATE [00:28:53]: this is all like backlash from the whole like bio-risk stuff, which is still figuring with the community very broadly. So not just technocrats is figuring out how to discuss risks of these models. I think that's great post on AI snake oil, which is like written by Sayesh Kapoor and Arvind at Princeton, which is really good, which is essentially like they've been really good. They've been getting the titles, right? So the title of this post was like AI safety is not a model property, which is like exactly the right prompt to get this across to people that might not be as involved in it. And it's really just that idea, which is like most of these issues are about how the models are used and like how they are allowed to be used on existing platforms or not. And like how I've been trying to frame this as what's going to, like, what is stupid for me to spend time on, but what's going to happen when there's a minor AI disaster. And like, how are they, how is the media going to react? How is the company involved going to react? And so little of it is about open versus closed. But I think that that's how the media will react to the first one. But like, it's so much more about existing infrastructure on the cybersecurity side, just how language models are weird. And like, it's just like the open versus closed is probably like the third or fourth point on that. And it's just like, it'll happen sometime. I think it's just like, I don't know how to transition us to a better future there of like getting, I think we need to better case studies of how language models are being used So if we understand a specific application, I think we could kind of do a better thing of understanding the risks associated with that application. It's all not grounded right now. It's just kind of all over the place.
TOM [00:30:36]: I feel like we also need better stories and better metaphors. The, the bio-risk stuff, that's a whole can of worms, which we could open up and smell and see how, you know, how ripe it is on this, on this episode if we want to, but I'm only bringing it up now because, and we see this, you know, like, yeah, there are these like fancy AI workshops or AI safety workshops that happen like at SMR in California and like other places around the world too. Right. And, and the reason they do that is not just because those are like nice venues where it's fun to go and like have coffee or dinner or whatever with other smart people. They do it because those particular venues have like storied histories in, you know, genetic policy and like how it is that we think about risks due to either nuclear weapons or due to like other supposedly or actually catastrophic technologies that we're trying to analogize back to. This is the reason why, and maybe this is finally changing now. I'm not sure yet. In fact, it might not be why people, if you ask normal people about AI risk, they still, if they close their eyes, see Terminator when you do that, that's just still the operative metaphor behind this stuff. And I'm sorry to say, you know, as like, as like techno, like people who are very close to the technology, we can tell ourselves that that's just the masses problem and that that doesn't, that that doesn't really matter. But the thing is, it really does matter because to an overwhelming degree, the stories that we tell ourselves and have told ourselves about risk over the past several decades or centuries, overwhelmingly determine how it is that when a crisis happens, any kind of policy response or media response is chosen to make sense of the situation. There's this need to make sense of what is going on and what always happens, whether it's a literal political revolution or a technological crisis or something else is people in positions of influence drawback from some bag of metaphors in their back pocket and just make headlines around them. And the headlines then become stories and the stories then become expectations and the expectations then become narratives and the narratives then become common sense. They just become the baseline.
NATE [00:33:00]: I would like to see some examples by which these narratives have been corrected because I feel like I'm trying to change the narrative and it's just such a losing battle. Like there are people that are trying to assess the narrative. Like I think the NTIA people are, they're like truly trying to assess the narrative on open versus closed to make these recommendations. But it's like there's a difference between changing the narrative and understanding the narrative. And I think one of them is even harder.
TOM [00:33:25]: I mean,
NATE [00:33:26]: I've said this before that openness doesn't have as good of stories to be told as existential risk. Like Terminator is a great story. What are we going to sell? It's like the meme. It's like the world. If open source AI existed and it's just like the utopia meme, like that's kind of the best we've got, but it's not easy for people to understand.
TOM [00:33:45]: I take a lot of inspiration historically from public health on this. So I don't think I've talked about this yet on podcasts, but there's a whole other side of my work still technically in AI. That's really more about rethinking AI risk and AI capabilities and AI infrastructure as public health problems, which is to say you understand them, not in terms of just whether all of humanity could get wiped out or not by them in some binary sense, but you understand them in terms of what is their concrete impact on populations of people over time? And how can we understand those impacts mechanistically? That's point one. And then point two is what constraints on the systems need to exist. So those impacts are either more observable or more actionable or preventable or can be more easily mitigated. And the thing is that's an old problem.
NATE [00:34:43]: Do you think we can actually get there in government structures? Do you think we can get there in the right amount of time? Cause I generally agree. We've seen this like the FAA and the FDA both have come about mostly because there was big issues. That's where I was going with this. So it might be like the minor disaster that I'm talking about is the thing that gets us off the ground.
TOM [00:35:05]: Another example is the history of the EPA on this, which is, you know, their environmental crises had been building for some time. I mean, you know, the famous, the famous catalyst is the Cuyahoga fire. So there's a river in Ohio. And there's a lot of Ohioans.
NATE [00:35:23]: I mean, that's a serious one.
TOM [00:35:24]: The Cuyahoga river literally caught fire. And again, now to be clear, that is objectively an environmental catastrophe, but what's really the reason it compelled people was how mind boggling it was that a river that big would catch fire, like genuinely disturbed people. And so it was, again, that kind of these underlying metaphors breaking down, that moved people to action. And so this was, you know, in the sixties leading up to 1970, you have the first earth day. The first earth day was a big fucking deal. Like cities shut down across the country, like Manhattan, there were protests and parades all day through the city.
NATE [00:36:09]: I don't even know. I just Googled it. Yeah. It's like, it started in 1970. That's so recently.
TOM [00:36:13]: Right. And it's also a lot more recent than maybe millennials are inclined to maybe assume. This is not like, it was really a transformation in consciousness. You know, this was not long after the whole earth catalog showed, you know, the full image of the earth taken from, from space. That was, that was a mind fuck for people because that had never been possible before to see everything on earth at one moment in, in one image. And it really did not for everybody, but it really did for many people open up this different understanding of our relationship with nature, which is that nature is not something that should be conserved or used or organized, but rather that we are the thing that is being organized and used by nature.
TOM [00:37:06]: And it's really like, we're just sort of suddenly reminded of our dependency on when these crises happen, but that's not a crisis because we've been irresponsibly managing nature. It's a crisis because it's showing how in feeble and dependent we are on ecosystems that we are really just one very small component in and they will just mess us up if we don't manage our own.
NATE [00:37:33]: What does this deal mean for AI? Like I, I, I don't,
TOM [00:37:37]: what it means is it's, it's all, it's all the link is feedback.
NATE [00:37:42]: I don't think you were saying that our society is about to be dominated by AI being so that we are small.
TOM [00:37:50]: I was actually about to make the point that the way that got handled was, so the EPA was created, the EPA was created as an independent agency in the federal government under the executive branch, basically to take a much more proactive rather than a reactive stance towards environmental policy. And there is this debate, this is not new, this has been happening for a few years now. Do we need like an FDA for social media? Do we need like an AI czar? Do we need a whatever? I'm not going, I'm not necessarily saying yes, that's what's coming, but what I am saying is the executive order on AI that came out in October already stipulated that every federal executive agency needs to have an AI
TOM [00:38:38]: lead in it, right? This is sort of, it's cutting across the table. There's whether it's health and human services, DOT, EPA, DOE, all the other ones, department of the interior. These are all really about how it is that unprecedentedly feedback laden data driven optimization procedures are going to, and are already changing our idea of what it means for there to be an environment or a coherent energy policy or a coherent transportation system or
TOM [00:39:16]: any other system that is being managed by one of those agencies. Now we're not yet at the point where we can even make sense of what I just said, but that's the kind of slow burning crisis that we're the, we're like the frog in that pot as it's just starting to simmer. So sooner or later we're going to either have to turn off the heat or get the fuck out of the pot. And what that means institutionally, I'm not sure, but I think it's tractable because again, what I'm describing is not itself new. There have been these transformations in consciousness and these new institutions that have eventually been created and codified in order to make sense of these transformations. But it does take time. It takes time because you're really birthing a new perspective. And so it's inevitable that it is going to follow in the wake of the technology disruption itself. It's going to be really how it is that we are able to reorganize and feel compelled morally to reorganize our own relationship with these systems. That's the moment when that's going to happen.
NATE [00:40:21]: I was going to ask you kind of a leading question, but I feel like I'm kind of already answering it. It's like, how does this relate to the one way door nature of open model weights, which is once they're released, you don't get them back. My kind of thinking on that is that open model weights will follow a progression of potential risks when they are applied. So it's like we're going to kind of ease into it. It's not like the next Lama four model is going to be AGI and we're all doomed. It's like, it's on a progression. So I think it's kind of set up for these things to happen. Like there is definitely randomness within the progression, which is how these models can be applied is somewhat sampling from a random process. And like, that could make it seem like it's a disproportionately bad, but I do think like it's grounded in these physical compute restrictions, which means like there it's bounded by some certain things. So it's, it's not sure thing that it'll be incremental, but it's, there are guard rails on it. That'll shape it towards being somewhat in this kind of steady price.
TOM [00:41:18]: I think it's also, there's a guard rail for a constraint, which is human nature. You know, we discussed this previously about like, why isn't there so much, why are there so many waifus pain generated by these models? That's not a natural feature.
NATE [00:41:34]: Do you want to know the name of our new Olmo model? I don't know if I sneak, you get to say it. So our 70 billion parameter model only has actually 69 billion parameters. So do you want to guess what the model name would be?
TOM [00:41:49]: That's propriety. Olmo. Nice.
NATE [00:41:52]: Okay.
TOM [00:41:53]: It's unofficial.
NATE [00:41:54]: This is the street name for a 69 billion parameter model. This matters. This matters, right? This is our,
TOM [00:42:02]: we have board goals on the line for adoption,
NATE [00:42:04]: so we need to make memes if we'd want to get our adoption goals hit. We are coming into,
TOM [00:42:10]: it's funny how cosmic my perspective is in this episode today. I mean, we are coming into a new relationship with ourselves as a data driven species. That is what is going on with large language models and generative AI by extension. And it's not surprising that in the early stages of that transition, we have a fundamentally puerile, adolescent prepubescent, and frankly kind of perverted relationship where, yeah, it's, whether it's waifus or whether it's those kinds of puns or it's Twitter, these are, these are immature ecosystems within which we are trying to make sense of what's happening to ourselves as we do it to ourselves. But I have a pragmatic confidence that we will grow into a relationship with these systems that is at least coherent and intelligible and that norms will emerge in the course of that, that will be more mature and more coherent. And I'm not necessarily saying good or bad. I think what I'm saying is kind of beyond that. It's more just, we will no longer be feeling like we are beholden to systems or models that
TOM [00:43:23]: lie beyond our understanding. Our understanding will become predicated on these models or systems in ways that we eventually discover to be empowering and, enabling of what we want rather than short circuiting what we want, that there will be a series of severe political repercussions that yes, we are still in the very early days of experience. It's like, let's loop this whole circle.
NATE [00:43:48]: How do these repercussions relate to this like apathy? Does this kind of apathy of people being less excited about open models change how these could potentially happen? My read of this situation, and this has been a through line in a lot of our conversations,
TOM [00:44:04]: is that the AI ecosystem, people who are in AI, people who build it, people who are very proximate to it, the culture is deeply dissociated where we kind of like thinking about these abstract models as if they're just going to, it's again, this is alchemy. It's just sort of like, there is just truth or possibility or, or frankly God that just sort of comes out of these systems just sort of automatically like ex Nilo from nothing to nothing, to nothing, to nothing, to nothing, to nothing, to nothing, to nothing, to nothing, to nothing. It just automatically like ex Nilo from nothing arrives some kind of intelligence. That's like,
NATE [00:44:50]: this is like the Latin saying for some new England college. I was surely like from nothing intelligence.
TOM [00:44:56]: Yeah. Well, from nothing, from nothing. It's a, it's the fifth element. It's this idea that, yeah, there's this earthly realm that we're composites or amalgams of, but there's this secret inner element that I can unlock if you just give me enough computer data or whatever it is. And the thing is that's, that's getting sublimated. And in the course of that, it's falling to earth. And a lot of people are realizing that that, that fantasy, that story that we told ourselves, but that honestly many of us are unconscious of is just not really true. It doesn't match up to the reality, which is that these fantasies are really just extensions of not having a living relationship with risk, vitality, what it means for a good system to behave as intended. There's a difference between just prompt engineering into something you don't have any mechanistic purchase on. Yeah. And actually having a good infrastructure. Yeah.
NATE [00:45:54]: More excited about my, it's a nice for me having covered this, like this is the first week I haven't written a blog post since like last August when I was on summer break. And it's like, it's nice that it coincides with the time where I'm like, okay, I'm excited about these things because I feel like I can now give a much more accurate representation of where things are by no longer just feeling like I'm constantly at this like Pareto that's getting pushed out and like barely hanging on. It's just not a place where you give good thinking and good analysis of the situation.
TOM [00:46:24]: It was a psychic cost. I imagine. Yeah. It sits in there too, because, because really like what, what we pay attention to is not a function. It's overwhelmingly not a function of just how good your eyesight is or how smart you are. It's overwhelmingly emotional. It's overwhelmingly about what drives you. What are you seeking? What is your goal? What is it that motivates you to look one way or another? That's what drives your basis for comparison. That's what drives why one thing is worth paying attention to versus some other thing. Yeah. And that's what's this, these deflated expectations that we're feeling now. That's, that's what that's about. It's the fact that it's no longer the rudder, the emotional rudder has kind of fallen off the boat, so to speak. And so we're getting inundated with models still, but it's no longer clear why we should care or whether we should care or what we should care about instead. And I think for me, I'm steady to the extent that that's not surprising to me. And it really just means we need to, yeah, you know, grow up a little bit, and think more about rather than just getting obsessed with the latest model, I should be more focused on what are the upcoming use cases that are going to become tangible soon? And how is it that we want those, we don't want those to happen in such a way that what ends up coming out of whatever model there may be is something that I would stand behind or not, or that I can speak about, or that I can say with substance, that's what openness would mean with respect to it or not. Yeah. And you're oriented. You're kind of, what does it mean to be oriented to those topics rather than just in some scalar sense on top of them is, I think, where we're approaching.
NATE [00:48:17]: Yeah. Welcome to the trough of disillusionment for all of you still listening.
TOM [00:48:24]: I am for one,
NATE [00:48:25]: happy to enter the trough. That's kind of where I feel like I'm settling in this.
TOM [00:48:30]: We're all pigs in the trough of disillusionment now.
NATE [00:48:36]: So just who will emerge first into the slope of enlightenment? Oh God, it's going to be Zuckerberg. Should we wrap this up with a little prayer to suck? I wish you were.
TOM [00:48:48]: You can leave.
NATE [00:48:49]: I'm sending him. Well, feelings for mama three. Good luck. Good luck, baby. Zuck rip. We do no editing, so we'll wrap up there. I'm okay with Zuckerberg. Some people really don't like him.
TOM [00:49:04]: I wish no ill will on anyone. Yeah. I try and maintain equanimity and there's always grounds for people to be actually, I can end, I can use my version of that prayer since I spent so much time in Appalachia. I've been to a lot of restaurants around the world, which is an amazing chain of restaurants, mainly in West Virginia. I doubt any of our listeners live in West Virginia. If you do, you should reach out, but you should definitely go there if you ever get the chance to. But there's a story where I grew up in Appalachia about the fence post turtle that feels relevant here, which is about somebody from the city who visits the country and he sees on a farm, a fence and on each of the fences, turtle sitting on top of the fence posts and he's confused. And so he calls out to the farmer and he says, Hey, what's this about? And the farmer is like, Oh, those are my fence post turtles. And the visitor says, yeah, I guess I can see that, but what the hell does that mean? Like what's going on with that? And the farmer says, well, here's the thing about a fence post turtle. He doesn't know how he got up there. He doesn't know how to get down. He maybe doesn't even remember how long he's been up there. He can only see what's right in front of him. And this is great.
NATE [00:50:19]: All he's going to,
TOM [00:50:20]: all that I've learned is important is to not put my finger in front of the snapping turtle or else he'll get bitten off. And the, the visitor is like, well, this is ridiculous. Like what, why do you put up with this? Like, what is it? You know, the farmers, you know, he kind of sighs and weary sigh. Shoulder visitor from the city. And he says, you know, you look like you got some fancy learning and some fancy money back where you come from, but I'll bet you that there's more fence post turtles where you come from than there are on my farm. And yeah, I, I think that, you know, I, I admire and I, I feel for the fence post turtles out there, but you know, don't, don't lose sight of, you know, what's important. Remember where you come from and how to get down.
NATE [00:51:12]: Sounds good to me. And we're, we're still grounded here. We're back after a few week hiatus. Thanks for listening. We'll, we'll catch you guys soon. And I'll talk to you soon with more furniture in the background.
TOM [00:51:25]: Bye everybody. Bye for now.
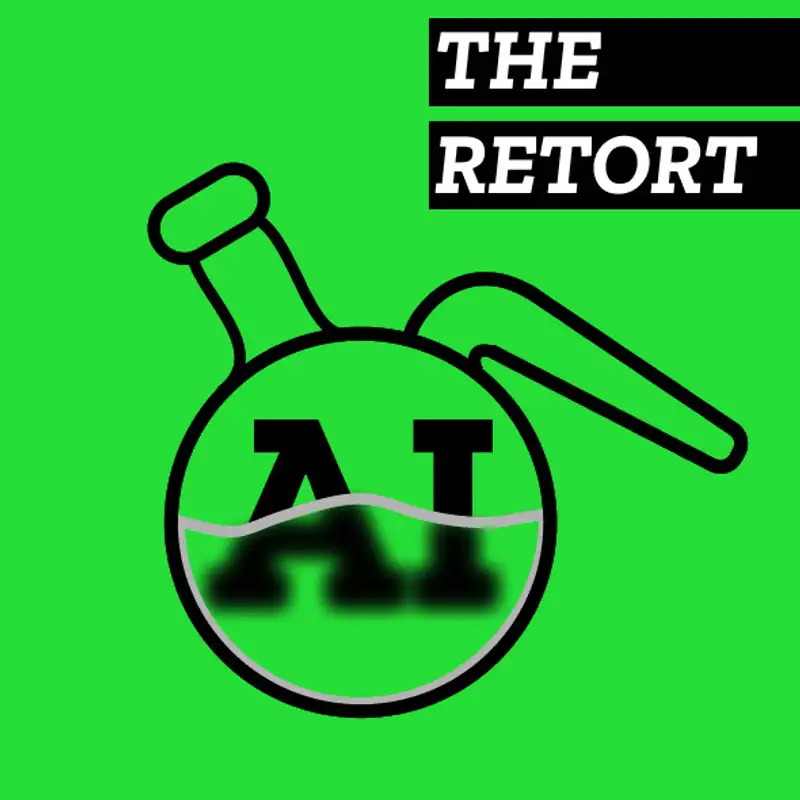