AI's Eras Tour: Performance, Trust, and Legitimacy
TOM [00:00:01]: Hi Nate. Hey Tom.
NATE [00:00:06]: Why did the generative AI avoid visiting Appalachia?
TOM [00:00:15]: Uh, I feel like there's some smoky mountain hallucination something joke right in front of me.
NATE [00:00:24]: Because every time I tried to generate a conversation about mountains, it got stuck in a loop about whether something was a hill or a mountain.
TOM [00:00:36]: In Rhode Island, we actually just have like bumps. They haven't even reached hill category.
NATE [00:00:43]: Yeah, Rhode Island's like super, super flat.
TOM [00:00:46]: Yeah, the landfill is the highest place in the state. It's the bay state. Yeah, the ocean state. So, welcome to The Retort, home of Rhode Island Facts, number one Rhode Island podcast, brought to you outside of Rhode Island. We don't pay taxes in Rhode Island. We're back. We're going to do a bit of a mixed grab bag. We're going to reintroduce Tom to the latest and greatest Jensen Wong News, leader of NVIDIA, quote unquote, the only stock you should buy if you're too online like me. But I only buy index funds anyways. We're going to talk about reward models and the thing that I released a bit just because the whole RLHF narrative is probably the most important, most interesting part of all of that rather than the details we found. And various current events that crossed our path. So I think we'll start with Jensen. So if you had to guess the last person to fill a large hockey stadium for a nighttime show on a stage with very expensive and coveted tickets for a singular personality in the Bay Area, who would it be with a bunch of people acting like teenagers, losing their mind, waiting for him to come out on stage? It's not Taylor Swift. It's Jensen Wong. That wasn't the greatest joke. But the jokes online were much better, essentially, because it's like the photos are so visceral, where it's literally like a dark stadium with people taking photos on their phone and visual effects. And then it's just Jensen Wong out on the stage with two computer chips. He's holding these little square chips that are these GPUs. And it's this long presentation. The fact that the Bay Area gets that excited for AI chips and hardware is very indicative of something with the AI mind share. You can literally take a photo of the Jensen Wong thing and the Taylor Swift tours, and they would look more similar than most things.
NATE [00:02:52]: Which stadium was it? Do you know?
TOM [00:02:55]: Whatever the San Jose hockey team is, I think. I don't know.
NATE [00:03:02]: Well, you know, everybody in AI, they're trying to skate to where the puck is going.
TOM [00:03:07]: I'm definitely impressed by why so many people wanted to go to GTC. Like what? It almost to the point where I'm like an online person that I felt like I was supposed to go. I was like, why the heck do I want to go to this computer trip trade show? It's not a trade show, but that's confused.
NATE [00:03:29]: Now that you've told me this story, there's a couple of newsletters that I've skimmed probably or got. I probably did see photos from this event, but I didn't understand exactly that it was the context of it. And I also didn't understand the Taylor Swift comparison. That doesn't make sense now.
TOM [00:03:50]: Even the Wall Street Journal and stuff was making this analogy. I didn't come up with this. That's how striking it is. If like boring places are making the analogy.
NATE [00:04:00]: I mean, part of it. Part of it's, I think, that we're still in this post-COVID moment, right? Where we're particularly prone to experience that collective effervescence of like a giant in-person gathering, especially if it's one that is not just a major sporting event. But it's like a moment or it's an era, to quote Taylor Swift, right? That we're still, I mean, no, all apologies to Taylor. But a lot of the reason her concert tour was such a phenomenon was that it was one of the first major concert tours after COVID when a lot of people felt comfortable doing that. It was amazing, right? But she cleared that benchmark, so to speak. So yeah, you can be happy for Jensen.
TOM [00:04:52]: It's almost like NVIDIA is becoming Apple-like. Because Apple also could do this.
NATE [00:04:57]: Oh, 100%.
TOM [00:04:58]: Apple chooses to select its audience and chooses to present its image in a different way. But not everyone could do what NVIDIA is now doing.
NATE [00:05:08]: I have to imagine, we're going to look back on this as a very idiosyncratic moment. When like, it's NVIDIA.
TOM [00:05:17]: It's like they were the most boring company. They just do graphics cards, yeah.
NATE [00:05:22]: I knew about them when I was in middle school, just because I was focused on whether like, oh, that card would play Diablo II better than whatever the fuck I was playing with at the time. And now they're considered, like they have this charisma. It's very funny to me. I have to imagine.
TOM [00:05:43]: Did you see Jensen's little mini speech at the Stanford Business School? No. He was essentially like, I wish hardship upon all of you. And I wish hardship upon all of my employees. Because you don't do good things without hardship. And most of you Stanford people have gotten into all of these good schools and you're a little entitled and that's okay. I was like, okay, he said it how it is. Like, you got to do hard things at some point in your life.
NATE [00:06:10]: He's not wrong. I didn't know he said that. That's just a weird, it's a funny thing to say.
TOM [00:06:16]: Yeah. It's all super funny. Like the NVIDIA moment is just, maybe we peaked. Maybe Jensen being Taylor Swift, maybe him like reaching that level for one day has shown that the AI hype has peaked. There's no higher target.
NATE [00:06:34]: I think the issue is like you and I are a little bit too young to have really had a formative moment in like the late 90s or like right before the dot-com boom. But I've heard stories, as you have, of like how fucking crazy it was, right? Yeah, this is basically like that, I think. We're getting to that moment. I have to imagine, yeah, we are in a bubble. Bubbles do burst. This one might just sort of deflate a little bit, though. Because, yeah, I think things have changed a little bit. It's just, again, I just want to return to this. It's just very funny that it's NVIDIA. That's still fucking funny to me. Like, that's just, it shows you so much, I think, about the moment. That it's this other, it's this completely.
TOM [00:07:24]: It's like the most boring company you work for. It's not boring in the sense that the work you're doing is impactful. But if you're on the tech market, people are like, go work at NVIDIA if you want a stable 9-to-5 tech job. Pretty remote-friendly, pretty good comp. Normal stuff.
NATE [00:07:40]: It's so revealing of where the excitement is, I guess, in the supply chain, too. So it's like NVIDIA does not design. It's not Apple in more than one sense, right? They don't design, you know, the interface.
TOM [00:08:00]: They don't design the thing. It's not a consumer thing. Like, how many of the people in that stadium have actually bought an NVIDIA product ever? How many people in the Apple stadium have bought an Apple product? Probably 99%.
NATE [00:08:15]: They're building the new alchemy equipment. They're like, you want to be the next. This is what it takes now. These are the flasks that you need. And we're building the next generation of those.
TOM [00:08:28]: Yeah. The other crazy happening is, did you see the Mustafa, Solo, whatever, asked the DeepMind? Saw that, yeah. Didn't he get kicked out of DeepMind for harassing people or being extremely toxic? That he founded his company, raised a billion dollars, and then got to go get a cushy job at Microsoft? I think Microsoft's strategic play makes the most sense, which is they're trying to diversify outside of open AI. So they also licensed inflections models. But like, I could go on a little 30-second walk to get his book, but I read like two pages because I was like, this is garbled.
NATE [00:09:08]: It's bad.
TOM [00:09:09]: It's just talking about bio-risk immediately. And all of that stuff has pretty much been debunked by like RAND and open AI themselves. So it's pretty outdated. I was like, I'm not going to read this.
NATE [00:09:21]: Again, we don't, at least I don't remember very many books about tech that came out in the late 90s or early 2000s. At least not ones that aged well.
TOM [00:09:30]: I have some founder advice and writer advice that go hand in hand. If you're going to be a writer, only write a book. This was told to me. Only write a book if you're willing to make that your top priority. And the same should probably go for a startup. Only found a company if you're willing to make that your top priority. And Mustafa did both.
TOM [00:09:50]: He was on a book tour and he found a startup. Look how that went.
NATE [00:09:59]: Yeah, it's a strange. Well, yeah. I mean, you have to admire Microsoft for being as cutthroat and strategic as it's been across the board. I wonder, like, think back. Others have reflected on this now, but it's early 2024 now. Try to reflect back on what Microsoft was like two years ago today. It's actually kind of hard for me to do that.
TOM [00:10:28]: Azure was kicking in. Azure was their big thing. And it was solid. But they've definitely jumped a bit.
NATE [00:10:38]: And now NVIDIA's in the mix, which is very odd.
TOM [00:10:41]: You look at the chart. NVIDIA is a bubble more than all. The AI stuff may or may not be a bubble, but the NVIDIA graph is obviously a bubble. If you're getting NVIDIA stock in your competition, sell that shit.
NATE [00:10:58]: Yeah, they'll have to be this shift, I think, away from just raw. Well, this is maybe also a bit of a transition to what you also do. The kind of reward stuff we can see. But their trust matters. Here, right. One way to understand, when does hype begin to die? Or when does the bubble begin to at least deflate, if not burst? It's when trust begins to palpably matter at least as much, if not more, than just the raw ability to stand in front of a stage and just have 10,000, 20,000, 50,000 people just scream your name.
TOM [00:11:31]: Yeah, I was writing about this just today, actually. I think evaluation used to be about performance, which is like researchers would take their thing and they would get a number out. That was a comparable number. But now it's also about performance, which is why government institutions matter. Sorry, I jumbled saying that. It's about trust and performance at the end of the day. It was performance, now trust. Government institutions offer abundance of trust because they don't have any shady incentives. While random startups have zero trust because they have the shadiest of incentives. And balancing that is not something that AI had to do in the past, which is part of why I think that AI, too, is cool, because it's a nonprofit, so it's somewhat trustworthy. But also they understand language models a little bit, so hopefully they can actually build evaluation tools that are useful. It sounds like good just advertising, so hopefully we could actually pull it off. But increasingly, trust is important, and it's kind of like reputation. It's a lot harder to build reputation than it is to burn it. Trust is like institutional reputation, I would guess. I'm interested if you have a kind of a way to describe the relationship between reputation and trust in this kind of AI space, because it seems like one should be a subset of the other or something like that.
NATE [00:12:53]: Well, two things come to mind, right? One, we've kind of, it's interesting to look back on, you know, we started doing these episodes in September, now it's March. And we've kind of experienced this transition over the course of it. I mean, we were discussing at the height of NeurIPS, neither of us were there physically. But at the height of NeurIPS, there was like at least one new model being released every day. And so I think part of this is just a kind of material fact, which is the market kind of just starts to get glutted or saturated with like just new models. And so just the attention economy almost just begins to dictate like, well, what's worth paying attention to? Who actually knows what they're talking about? Or what are the organizations or companies that I would, yeah, trust more than others to weigh in on whatever's going on right now, right? So I think it's partly just a kind of fact of like, you can only look at so much, understand so much at any one moment. And when people just ride performance into the ground, there has to just be some other basis on which to evaluate. And that just happens to be trust, because we need something else. So that's one side of it. The other side of it, I don't know if this answers your specific question, but there's another dimension of this too. Maybe this is also where government comes in. So there's performance, absolutely. There's trust, which we are now maybe just on the cusp of grappling with as an independent dimension of evaluation. And then I would argue there's at least one more, which is legitimacy. So you can trust something because it works as intended, or it has worked in the past. So there's a kind of just basis on which you're like, sure, I would keep looking to this thing. But, you know, in political theory, and also I think in governance, there's this issue of like, who has authority to speak on some topic? Or who has authority to define what counts as a good standard or not? And, you know, nominally, maybe this is pushing a little bit. I mean, I'm interested for your thoughts on this of like, what is it that distinguishes something like AI2 from something like NIST? Because NIST is a government entity.
TOM [00:15:21]: What differentiates those two from a new nonprofit that's founded this year that wants to nominally do evaluation? That's another thing. There are more nonprofits coming. And I think maybe legitimacy is where that will come in, which is like the longevity of doing it. I don't know if I have a good answer between the difference between trust and legitimacy. Because everyone talks about the breakdown of trust in our institutions, and the institutions are mostly legitimate. And so I'm trying to figure out if legitimacy is a prerequisite for trust, or if it's something slightly different. Because they're definitely very closely tied. And I think it's important to understand what that means when more government institutions are talking about AI. So for example, there's an NTIA request for comment out right now. And it's like, is it the National Telecommunications and Information Administration? These government agencies have, this is under Gina Raimondo, the Department of Commerce. It probably has like a $10 to $100 million per year budget. It's smaller than the Allen Institute probably. And what they can do is they could comment on how other federal agencies and how companies should perceive AI and start thinking about AI. That's the breadth of what they're going to do. They're not going to build powerful AI systems. And what does that mean for their trust and legitimacy? Do you have more trust because you're actually doing the thing? They're pretty legitimate as any old government institution in my mind. And if we could use this to kind of create a mental model for what will matter in government agencies talking about AI, I think that's pretty important. And how policy will unfold. Because at these policy events, I see more policy unfolding from these agencies rather than regulation, which is not surprising. But it matters because it's kind of a different room to be in. Right.
NATE [00:17:31]: Part of it is who has mandates to do what. Right. So a lot of what the executive order on AI was that came out in the fall was, I mean, I think people who work in AI are just not sensitive to this, which it's fine. It's not your job. But like just to like a little bit of constitutional one on one on one. Right. The executive order was not a law.
TOM [00:17:54]: It was not. I know this.
NATE [00:17:56]: Thankfully, here's what I mean by that. There's a few there's a few reasons that's important. First of all, Congress did not pass it. Secondly, it did not create any new mandates on behalf of like any existing. It didn't create any new agencies. And even amongst the agencies that already exist, it did not give them any additional powers that they did not already have. So all the executive order did was clarify how the existing mandates that executive agencies have are relevant or applicable to the context of recent developments in AI. That's still enormously significant. It's an enormously significant document. And actually, as we previously discussed, one that I was mostly pretty impressed by, actually, for several reasons. But it's literally just the effort to further kind of sharpen or precisify what NIST's job is with respect to these new capabilities or other agencies, for that matter, because there's several agencies that it discusses. But those agencies are really just parts of the executive branch. They're just sort of organs that historically differentiated in response to recent developments. And it made sense for the White House to be like, rather than me having to make up a new environmental policy every time there's a new president, we should just have an EPA. That's staffed by experts that act in coordination with my policies or with my priorities, with my whatever, whatever. That's all it is. So it's really a kind of miracle of the federal government that this works. But it's really, you should think of the executive order as sort of like, I sort of think of it analogous to putting a respirator on somebody or shocking somebody's chest to get their heart to start beating. It doesn't put a heart in the body. It's just like restoring a shock to the system to awaken it, to alert it, to direct its energies and its attention towards a problem and apply all of its existing standards and procedures and staff to that problem.
TOM [00:20:26]: I have two related things. I haven't answered the question about the difference between trust and legitimacy. I'm kind of becoming a downer on that. I think the first thing is a downer on the taxonomy. The topic is important. The other thing is about trust and legitimacy of organizations in the space is the UK AI safety org has primarily been funded by big tech companies. And now the US is starting one, which whether or not you get government funding or big tech companies is a thing. And it's multi-level. So even if you get it as an unrestricted gift in the first thing, it's like, then who are you beholden to to ask for money in the future and how that might impact your policies is the type of things that these things worry about. I was at this policy event with a bunch of different companies and actual policymakers. Someone was in the room who was in the EU legislative body for 10 years. So it was actually policy people trying to make sense of the nonsense that I spouted out of my mouth. And that was a big focus point for them. It's like the US Safety Institute can be different by getting more neutral types of funding, which is very real. But I don't know. I just don't know how to fix it into our taxonomy. And that's the type of thing that will define trust. The Allen Institute's money, I think, is pretty well known that it's from Allen. It's Allen. It's the Allen name. I'll say that's all I need to say. But if it's like another evaluation thing is funded by like Eleazar Youkowsky, what does that mean for their trust and legitimacy? So this all goes back to the whole brand conversation. I'm talking about Jensen Huang. And it just constantly gives and takes. It's a constant finding of equilibrium.
NATE [00:22:19]: I agree. It's definitely finding of an equilibrium. To comment briefly on that. I mean, assuming what you just said is all true with respect to the UK Safety Institute vis-a-vis the potential or impending US Safety Institute. Yeah. How would I grok it for me? And I suspect for many other people is if one of those bodies is overwhelmingly funded by big tech and one of those bodies is not overwhelmingly funded by big tech, but they are both under the respective governments of the countries in question. In theory, they would both be legitimate. But I would trust one of them way less than the other one, depending on how much time goes by.
TOM [00:23:11]: It's kind of like the difference between credentials and reputation. Where it's like if you take any old Berkeley grad student off the street, they all have the same potential. But if one of them goes to work at a quant bank, you're not going to trust them on matters of AI safety or something like AI society type things.
NATE [00:23:27]: I might even put it a bit stronger, which is it's the difference between having credentials and being a source of accreditation. So a university professor on AI, Stuart Russell, for example, is generally a pretty trusted expert.
TOM [00:23:49]: Yeah, that's not a hot take. He's like one of the most trusted voices in all these rooms.
NATE [00:23:54]: I think he even prides himself on that to a specific degree. Anyway, let's say that he is.
TOM [00:24:02]: Do you have the other side? I want to see what your other example is.
NATE [00:24:07]: Oh, well, where I was going with that was to say, sure, Stuart's trusted, and I think he has reason to be. But that's because he I mean, frankly, that's because he came up at an accredited university. The university was accredited by the US government to be a university. Universities that can't get accredited are they don't they don't last. This is a major issue with the new university that they're trying to start in Austin. It's called like I'm going to I should actually look this up.
TOM [00:24:37]: Is it literally like the stars and their mascots can be the stars and stripes or something?
NATE [00:24:42]: Uh, that's news to me, but it will surprise me. So I believe that's right. So I just I'm sorry to our listeners. I just had to make sure I had this right. So it's not UT Austin, which is a serious accredited university.
TOM [00:24:58]: It is a great school.
NATE [00:25:00]: Yeah. Go Longhorns. We're fans. It's fine. I'm speaking about the University of Austin, which is a different entity. And I mean, yeah, from everything that I've read about it, I've been curious. And so I've been following it a little bit. It's it's this effort to create a university that is sort of, in some ways, a callback to what universities, quote unquote, used to be before they became, you know, however you want to put it, woke, excessively focused on optics or rankings.
TOM [00:25:39]: Well, sure.
NATE [00:25:40]: Yeah. There are different ways of describing this irony, which is that in the minds of some people, especially elite universities, have become primarily sources of status and secondarily or even much less than secondarily sources of actual learning or independent inquiry, independent knowledge inquiry. So this University of Austin, in part, has been pushed as an answer to that. From what I understand, its major roadblock, despite having raised a fair bit of money and having some very prominent big wig academics stand behind it, is it's having trouble getting accredited. In other words, it's having trouble convincing the government that if you spend four years at it taking enough classes, you actually have a degree that others should be like that's a degree in that field. And so that matters, right? Accreditation is about are you a legitimate university? And that's conferred by organizations that are already legitimate, namely governments or states.
TOM [00:26:47]: Do you think there needs to be accreditation of like your AI evaluator? 100 percent.
NATE [00:26:51]: Absolutely. 100 percent. Yeah. You need you need to have in the long term. Absolutely. I think I think so much when you zoom out from where we are right now, five, 10, 20 years in the future. We're we're in this transition period of like it's not quite the Wild West anymore, but you can still be NVIDIA and fill a stadium like because basically just because you can. So we need to transition to who, again, think about the Wild West, like who's the sheriff? Who's the postmaster general? Like who's the all these roles need to get filled and they can't be filled by randos. They have to be filled by people who seem to be good enough at the job, trustworthy enough to regularly perform it. And are seen and I'm sorry to say we do technically still live in a democracy, but one which popular sovereignty or consensus is able to form that like, yes, that is the person who it makes sense to do that job. And that's a that's a that is a distinct axis here. And so, yeah, ultimately, we are going to see I think we will see this. But I think also, I mean, I'm not an accelerationist, but insofar as I am one, maybe this is the one direction of it is I want there to be a faster transition to how it is that authority gets minted, how it is that the conferral of authority can happen so that we can skip past some of the chaos to a landscape of authentic. Legitimacy as the bedrock on which trust can can emerge and coalesce.
TOM [00:28:31]: I have a rough transition. I've seen your e-reg. How do you what is e-reg stance on open source AI?
NATE [00:28:42]: What it wait what it was describing.
TOM [00:28:45]: Yeah, what is like what is like does it I feel like it's probably agnostic, but it's leading into another more interesting point that I have to make.
NATE [00:28:53]: And sorry, on open AI is like open AI.
TOM [00:28:56]: It's a topic, not a company. It like does more openness help you make help those institutions get formed? I think it probably doesn't much on principle, but big companies might slow it down because big companies slow things down.
NATE [00:29:10]: Openness as like openness with a capital O as like a value, I think, is a necessary but not sufficient condition. And also, it's probably only necessary at certain stages of this process. I'm more concerned. I think I've made this clear on previous episodes. I'm generally because because this the reason I'm emphasizing legitimacy so much. It's not that, you know, it's not all that matters. Obviously, the models have to work performance still matters. But legitimacy is important because legitimacy is ultimately about accountability. And transparency and openness is for me, it matters really only insofar as third parties can then be in a position to meaningfully assess whatever has been claimed or released or deployed. That's the reason it matters.
TOM [00:30:11]: This was leading because essentially openness is like one of the worst words right now because everyone just uses it, but no one knows what it means. So in this kind of policy event that I was at, one of our subgroups, one of the leader of the subgroup came up with this definition. And it's William Isaac, a friend of the pod at DeepMind. He came up with the definition for what people mean by openness into three terms. So he bucketed like openness as a value into disclosure, which is like the details of their processing. Accessibility, which is that people have the tools to use it. So like Hugging Face would fit into that. GPUs being the right size for GPUs. And then availability, which is kind of like a yes, no, if broad people have access, which I really like. Because now we can talk about what different people are doing for openness. Because like Grok, like Grok open sourcing it is, yes, it is very available, but there's very little disclosure and there's very little accessibility. Which means that it doesn't really shift the narrative at all. But it's just nice to be able to inform and like actually discuss these things.
NATE [00:31:15]: Yeah, we haven't talked about Grok yet. I was going to come in with that and say that now that Grok has been made, quote unquote, open source. It's sort of like when Obama finally made, he himself made the Thanks Obama joke. There's like a video he came out with. He's like, I think, if I remember correctly, he's trying to dunk a chocolate chip cookie into a glass of milk, but the cookie's too wide. And he just sighs and looks at it and says, thanks Obama. And so I think there was a kind of collective agreement on the internet that no one could make that joke anymore because it had reached its source. So yeah, I think there's a certain, we've clearly, it's important. I think the fact that Musk did that is clearly an important step in the direction of like we need to significantly level up our semantics around what openness means and what it doesn't mean.
TOM [00:32:11]: Yeah. Yeah. I mean, just because he's involved, it makes it worse. Like he brings eyes. He's like the little like spotlight beam. And if he's on an issue, it has a lot more attention. And when there's an attention on something that's a problem of like whether or not it's just easy to describe, it just makes it worse. So like, I think it's good.
NATE [00:32:34]: But picking up what you said, the taxonomy you gave, because what was it? Disclosure, access, and what was the third thing?
TOM [00:32:41]: And availability. I think they're like a good working definition. Kind of like I was trying to come up with another definition of like what an open source LLM is. Like this is better than what we got, but I don't think it's perfect. It's not.
NATE [00:32:53]: It's certainly progress. I mean, I'm actually organizing this workshop on disclosure for RLHF at the academy. Not because I've been speaking to William or something, but just because I think even I sensed that like.
TOM [00:33:09]: He came up with this on the spot. So I like asked him, I was like, is this something you've been percolating? And he was like, no, it just seemed right for our conversations. What is it?
NATE [00:33:17]: Right. Well, what is it that's actually. Look, I mean, this is fair. Look, if you're trying to get from alchemy to chemistry, how do you do that? You have to like understand like, well, beyond just transmutation. What does it actually mean when you're doing an experiment and you're allowing certain variables or reagents to interact in a controlled setting and disclosure? I made that association because it's almost literally like, yeah, how, like literally what valves am I twisting so that this chemical can interact with this other one?
TOM [00:33:50]: And I think the nuance thing for RLHF is like, we might not have the mechanisms for disclosure that we need to actually understand things. And if you're trying to do this step of like doing openness and doing transparency and accountability, like in order, you will see that you're limited on one of these things if it's not happening. You might have complete availability and still not make any progress on auditing RLHF because the disclosure mechanisms aren't good enough or something like that.
NATE [00:34:18]: And that is very much going to be a theme of what I imagine we will discuss at this workshop. And that's why it's interesting even now as I reflect on what my politics are on these questions. I still feel like in many ways, if you're acting in good faith and you really even want to understand what the fuck's going on, not even enough is disclosed to even really get a clear sense of what is possible or impossible with these models.
TOM [00:34:46]: I mean, that's the whole motivation of the reward model thing is whether or not we're going to discuss it. But for people that don't know, I feel like most of our listeners probably read my blog, so we'll see it self-serving. But it just means our pod audience is small and loyal and we appreciate you. But I released a benchmark for reward models called RewardBench. And there is a data set that you could get a score on. We did the thing, if you release an evaluation score, a benchmark, it needs to have one number so that people can compare their models. It needs to just have that basic stuff. But more of it is just tooling to have people to release reward models. There's only 10 reward models on all of HuggingFace that match the description of what RLHF is, where you have this classifier that returns a scalar value. The fact that there's only 10 is so broken in terms of the availability to study this technology. And there's a lot of these models trained with DPO, which is different. And we compared them as well. But the focal point that there are literally just 10 models in this general RLHF notion is so beyond bizarre. And that's what I want to fix. And it's just silly. I did skim it.
NATE [00:35:57]: I saw Berkeley is doing quite well. That amused me.
TOM [00:36:01]: Go Bears. Go Bears. We kind of cooked our books because we were timing our releases. Their top model was only released today. So we were doing some good old Berkeley collusion to make each other look good.
NATE [00:36:16]: So there's an example of what's the most important thing that you did there. Is it the specific rankings of those models? Or is it the standard that you're putting forward? Because that's really what you're introducing.
TOM [00:36:32]: Yeah, it's the standard. It's that new mechanism disclosure. It's like you can disclose this about your reward models and no one has disclosed anything. And it's not a lot of information. It's not that telling. But that's what it is. And we're working on trying to get this information from tech companies and stuff, which is a slow grind.
NATE [00:36:50]: I feel very strongly. The common denominator here is the number of interfaces off of which we're trying to set policy or have informed conversations about evaluation of models or really anything in AI is a drop in the bucket less than what there needs to be in terms of the infrastructure. So literally, just again, the fact of so much of this is vibes driven. So what are the interfaces that matter in AI? Twitter would have to be like in the top five or top three of just like that's crazy. But that is the world that we live in. That is, in fact, the way it is right now. I feel I have hope that eventually that will change and we will have either some fully agreed upon consensus driven leaderboard of what really matters. And that will be to some degree a moving target. I mean, certainly the models on it will be a moving target, but maybe the interface itself will eventually stabilize and the ecosystem will have matured so that everybody at least agrees on what companies are in fact competing over and why that matters. And that there's, again, this kind of like sense of legitimacy around that of like, yes, these are the things that are worth competing on or not competing on. And then within that, I'm the one you should trust or my model is the one that's most trustworthy or whatever it is. So, yeah, it's kind of beautiful in a way.
TOM [00:38:21]: Do you think a for profit institution could fill this void? Like at a structural and what reputation means? Because I think there's such a need that the big companies would be willing to pay for it. But I don't know if it's like they just pay a nonprofit and they do it. Like a nonprofit doesn't necessarily have to be free. Like they could cover their costs with the payment or something.
NATE [00:38:42]: I believe that a for profit company or corporation could do this. Yes. I mean, we know that's true historically. We know that there are public or there are private companies that effectively act as public utilities that make money. But they do it in a way that is, again, rigorously monitored and whatnot because they have to fulfill certain commitments. But there's no reason in principle why you can't also make money and do that. I think it's really a testament to how brazenly off base the incentives are in A.I. That that's not what's happening right now. Right. I mean, the fact of like. Yeah, I think what meta what Facebook in particular is is doing with its social media platform is a joke with respect to responsibility. I mean, it's across.
TOM [00:39:34]: So you mean like the serving that they do?
NATE [00:39:36]: Yeah. I mean, the fact that there's this pretense to protect users, they're not they're not doing that. I'm sorry. I'm sorry.
TOM [00:39:47]: What is this? They're just they're just not. It's like you have and it needs to be the next year, seven managers in five years. They have seven trust and safety teams in five years.
NATE [00:39:56]: It needs to be said that it's I'm sorry. It's just no, you can't you cannot manage a company of that scale that makes money in that way. That intensely doing what it does to users as often as it does all over the world with that number of people do like monitoring it. You just you can't.
TOM [00:40:18]: You can't.
NATE [00:40:20]: And it's important because then what that means is that's the reason why the responsibility stuff has just been stuck in Kabuki theater. Especially by Facebook, but more broadly theater.
TOM [00:40:29]: Do people know it? Do our listeners know what that is?
NATE [00:40:31]: That's I understand.
TOM [00:40:33]: I feel like I've heard it before, but it's just funny.
NATE [00:40:36]: It is a metaphor, but it's also literally a style of theater, which is why it's funny. We can put a link to Kabuki theater in the show. Yeah, it's an SF.
TOM [00:40:45]: It's literally an SF. Yeah, I mean, you'd be better.
NATE [00:40:49]: It'd be more edifying for our listeners to actually go to a live performance of actual Kabuki theater by professionals. Then watch Mark Zuckerberg apologize to parents whose children have killed themselves in front of the Senate.
TOM [00:41:01]: Yeah, that whole thing is rough. It's like the politics and everything of it are just the politics, the technology, the world we're at is just all rough. We're in this weird transition and AI is making it faster, maybe worse.
NATE [00:41:22]: I think you have to embrace the transition. You have to embrace the indeterminacy of this.
TOM [00:41:27]: It's funny. I'm reading The Three-Body Problem in the second book. It's all they know the aliens are coming, but it's 400 years away. I'm very early on, so it's not really a spoiler. It's like, okay, that's a big transition. It's a multi-generation one, but it's honestly potentially similar. It's like AGI-ish things are coming in a few hundred years. What is AI going to look like in 200 years?
NATE [00:41:53]: Or five years, if you believe Eugene. That's what I saw.
TOM [00:41:57]: It depends on the definition. I'm fine with the definition of GPT-4 being AGI, but a lot of people think AGI needs to have a level of autonomy that GPT-4 does not have. People are forcing entity status and autonomy onto AGI, which I don't understand why that is necessary. That's this whole AI story with agents and independence and personhood that people assign to AI. But I think that AI as a powerful tool in a general fashion, artificial general intelligence, that's what the word says. It is more of a storytelling to require otherwise. Maybe I'll start beating that drum. Maybe I'll write a blog post that's GPT-4 is AGI. Really stir the people up. Seems like a great thing.
NATE [00:42:46]: I may not ride that with you, but I take your point. I think it's an important point. I think what keeps me excited, because this is important, we need to confront what an abyss we're staring into here. And maybe we're really only doing it now because it's no longer just hypothetical forecasting. We literally now have Eugene filling stadiums in San Jose off of these chips and claiming these things. These are no longer just abstractions. We're looking for ways to ground the abstractions. But when we look down at our feet, we're like Wile E. Coyote and we just see this sheer drop. And we're not sure when or how we're going to fall or how far or how many of us are going to perish or something in the course of it.
TOM [00:43:36]: I agree totally. What do you think Mustafa's PDOOM is? Probably zero. He's in it for the money.
NATE [00:43:45]: His actions speak to somebody who has it lower than Lena Kahn, certainly.
TOM [00:43:51]: Would you say hers was?
NATE [00:43:53]: Hers was really high.
TOM [00:43:55]: It was 15%. If your PDOOM is not lower than Lena Kahn, you should be doing only AI safety research all the time.
NATE [00:44:02]: We should call that the Lena Kahn litmus test or something.
TOM [00:44:05]: Probably. We'll come up with that. I can't believe that happened.
NATE [00:44:13]: No, but I do want to end on this, at least for me. What gives me hope is that, sure, we debate what AGI is. Well, that's an opportunity for us to become much more rigorous and clear about what we mean by intelligence.
TOM [00:44:27]: Yeah, I've really liked that short exchange. That clarified a lot for me on the importance of agency and storytelling in it. I hadn't put that together.
NATE [00:44:36]: Agency versus, is intelligence a faculty or is it a feature of agency?
TOM [00:44:42]: It's like the deep time versus open AI debate. Open AI has all been building generative tools and deep time has been obsessed with RL. Those are probably the two biggest names in AI, historically.
NATE [00:44:53]: Yes, I have long sensed that this matters. It is what initially attracted me to the field. In a way, I'm excited that the basic unresolved philosophical stakes of what are really ancient concepts and questions are now beginning to be confronted. It's not because we've gotten smarter. I think it's just because we've kind of painted ourselves into a corner where we're now building things that we don't understand and don't know how to evaluate. We have to now come to decisions about this.
TOM [00:45:26]: Yeah, I agree. I agree. It's going to be a fascinating year. We're zero to two years away from a powerful AI agent of some type in my brain.
NATE [00:45:36]: It's crazy. Yes, it's crazy. Some kind of moving DDoS attack that as we attack it, it adapts. Well, I mean, yeah, now we're in a forecasting world, except it's actually, you can imagine it now. It's a remarkable time.
TOM [00:45:53]: Yeah. So that's fun. That was a bit of a mailbag, or a self-fulfilled mailbag. We also take questions if you email mail at retortai.com. You can send us your five-star reviews if you're too timid to put them into Apple or whatever platform you listen to.
NATE [00:46:09]: Or send us jokes.
TOM [00:46:11]: Yeah, we can do a crowdsourced joke. Make the people decide. I mean, the New Yorker did that with their cartoons.
NATE [00:46:17]: They're like, send us in captions on our cartoons. That was like a major boon for their response for it. Yeah, you can try that.
TOM [00:46:26]: Cool. Sounds good.
NATE [00:46:30]: Nice catching up. Sounds good for this week. Yeah, nice catching up. We're both very busy, but happy to make time for our listeners and for continuing these conversations, because it's going to be a wild ride. It's been a while. It's going to get weirder. We'll be there for it.
TOM [00:46:47]: Sounds good. Bye for now.
NATE [00:46:51]: Bye for now.
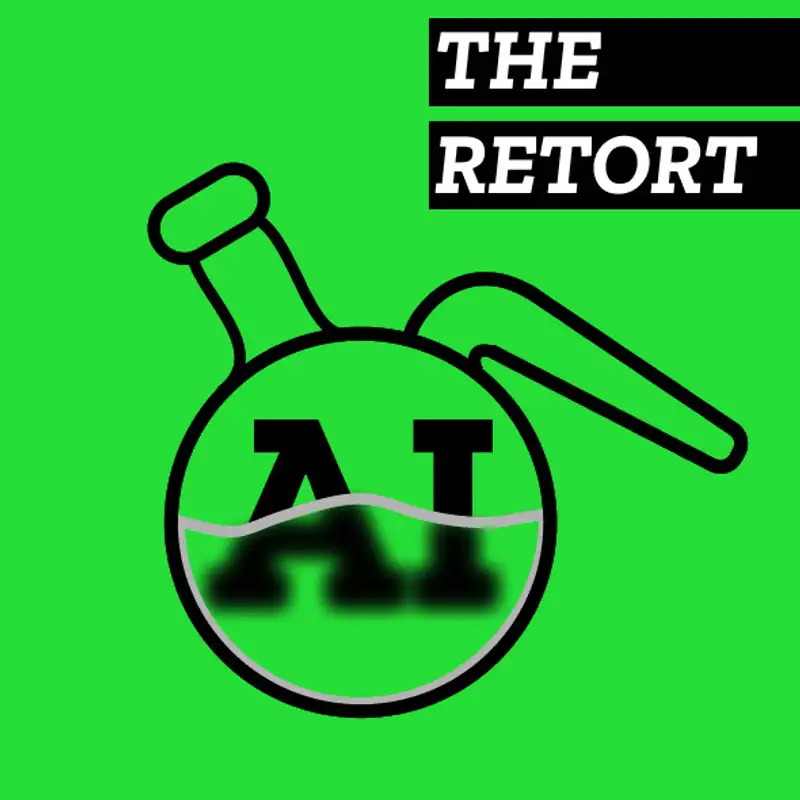